How Generative AI is Transforming Application Modernization
Per the trends suggested by Red Hat, modernization is among the topmost funding priorities (45%) for global businesses - all thanks to the promises of better digital experience, cloud-native, and quicker time to market. Generative AI can fulfill these promises by automating legacy system updates, enhancing user interfaces, facilitating cloud migration, and expediting software delivery. Moreover, the marriage of application modernization and generative AI will directly impact data availability, privacy, security, and other concerns. It’s not a surprise that organizations are already looking forward to an unprecedented acceleration in their modernization programs by leveraging generative AI.
Therefore, this blog will help you explore application modernization and find the appropriate place for generative AI. We will set the context for the immediate need for generative AI in modernization efforts and discuss what practical advances this union brings.
Before we jump into the discussion, you must check out our latest webinar where our CTO, Haresh Kumbhani, will be talking in detail about leveraging generative AI for modernization
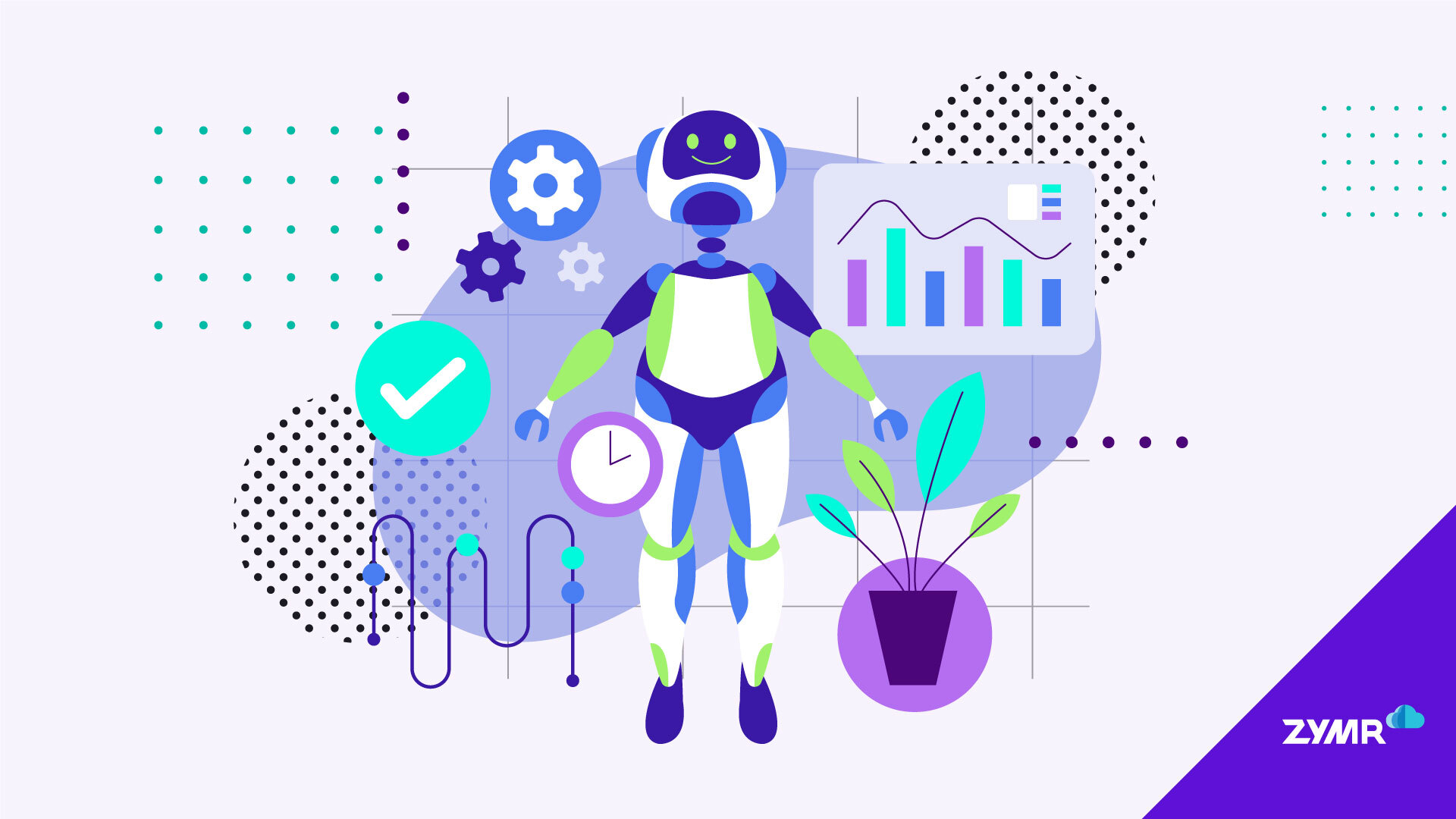
What Generative AI Brings for Application Modernization
Over 50% of enterprise GenAI models will be industry or business-function-specific by 2027. Such Gartner predictions urge decision-makers to anticipate a rising demand for tailored solutions. Thanks to generative AI, this is one of the many precursors that point to transformative shifts in application modernization.
Here’s what Gen AI brings to the table.
- Edge Computing Integration: Generative AI plays a crucial role in integrating legacy applications with edge computing systems by automating the adaptation of software architectures and protocols to suit edge environments. Through sophisticated algorithms and model training, Generative AI assists in generating lightweight and efficient code optimized for edge devices, enhancing the performance of applications in distributed computing environments. This automation enables seamless interaction between legacy systems and edge devices, facilitating real-time data processing, reducing latency, and improving responsiveness for critical applications.
- Hybrid Cloud Environments: In the context of modernizing legacy applications for hybrid cloud environments, Generative AI streamlines the migration and integration process by automating tasks such as workload placement, data synchronization, and network configuration. By leveraging Generative AI, organizations can generate cloud-native architectures and deployment models tailored for hybrid cloud setups, ensuring compatibility, scalability, and flexibility across on-premises and cloud infrastructure. This automation minimizes the complexity and even aids with security testing for cloud-native applications.
- Cybersecurity Enhancement: Generative AI is also a powerful ally for cloud security posture management (CSPM), essential for modernized applications. By automating the identification and mitigation of vulnerabilities, it can neutralize the threats posed by legacy systems. Through advanced threat modeling and risk assessment, Generative AI assists in generating secure coding practices, encryption mechanisms, and access controls, strengthening applications' resilience against cyber attacks and data breaches. This automation enables organizations to proactively address security gaps and compliance requirements, ensuring the integrity and confidentiality of sensitive information in modernized applications.
- Digital Twin Development: In the realm of digital twin development for legacy systems, Generative AI facilitates the creation of virtual replicas and simulation environments through automated modeling and simulation techniques. By leveraging Generative AI, organizations can generate digital twin models that accurately represent the behavior and performance of legacy applications, enabling predictive maintenance, optimization, and analysis. This automation accelerates the adoption of digital twin technology, empowering organizations to monitor, analyze, and make data-driven decisions based on insights derived from virtual representations of physical assets and processes.
- Industry-specific Applications: Generative AI enables the development of industry-specific applications tailored to the unique requirements and challenges of different sectors such as healthcare, finance, manufacturing, and transportation. Through domain-specific model training and customization, Generative AI assists in generating application features, functionalities, and workflows optimized for specific industries, enhancing productivity, efficiency, and innovation. By leveraging Generative AI, organizations can address domain-specific needs, compliance standards, and regulatory requirements, delivering tailored solutions that meet the evolving demands of customers and stakeholders in various sectors.
- Human-Machine Collaboration: Generative AI promotes human-machine collaboration in application modernization by automating repetitive tasks and augmenting human capabilities with AI-driven insights and recommendations. By providing developers, engineers, and domain experts with AI-generated models, code snippets, and design patterns, Generative AI facilitates more effective collaboration and knowledge sharing. This automation empowers teams to accelerate the development and deployment of modernized applications while focusing on higher-level tasks and strategic initiatives, fostering innovation, creativity, and continuous improvement.
- Continuous Integration/Continuous Deployment (CI/CD): Generative AI facilitates continuous integration and continuous deployment practices for modernized applications by automating the build, test, and deployment pipeline. Through the generation of CI/CD workflows, scripts, and configurations tailored to the specific requirements of legacy systems, Generative AI streamlines the software delivery process, reducing manual intervention and accelerating time-to-market. This automation enables organizations to iterate more rapidly, gather feedback, and deliver value to end-users and stakeholders reliably and efficiently, ultimately driving innovation and competitive advantage.
Ensuring Operationally Optimized Modernization
The promises of generative AI discussed above bear a deep operational impact on digital ecosystems. Even for domain-specific and industry-tailored modernization strategies, this operational effect is necessary. Without offering a transformation that hits at the cultural level, businesses cannot trust the feasibility of generative AI for modernization. Therefore, let us now try to understand the impact of generative AI at an operational and cultural level on businesses.
- Efficiency in Legacy Analysis: Generative AI can help streamline the analysis of legacy systems, which is crucial now more than ever. It automates the extraction of insights from complex codebases and documentation. Instead of manual analysis, which is time-consuming and error-prone, Generative AI can help rapidly identify dependencies, business processes, and architecture patterns, enabling a more thorough understanding of the legacy environment in a shorter time frame.
- Velocity in Modernization: Time-to-market is a critical metric in today’s digital economy. Generative AI can help accelerate modernization by automating repetitive tasks such as code generation, documentation, and test case creation. Tasks that would typically take weeks or months can be completed in a fraction of the time, allowing organizations to execute their modernization initiatives with higher speed and agility.
- Optimization of Resource Utilization: The current competitive landscape demands maximum productivity in given resources. Generative AI can help optimize resource utilization by maximizing the productivity of development teams. By automating mundane tasks, developers can focus their efforts on high-value activities such as architectural design and problem-solving, leading to more efficient use of time and resources throughout the modernization project.
- Performance Optimization: Domain-specific Generative AI models can be optimized for the unique characteristics and requirements of a particular industry or business function. By tailoring AI models to specific domains, organizations can achieve higher performance and accuracy in their applications, leading to better decision-making and outcomes.
- Improved Interpretability and Trust: Domain-specific GenAI models can also be easily interpreted compared to complex, black-box models. This interpretability is critical, especially in regulated industries where transparency and accountability are essential. By using models that are tailored to their domain, organizations can enhance trust and confidence in AI-driven decision-making processes.
- Complexity of Legacy Systems: The complexity of legacy systems poses significant challenges during modernization efforts, including understanding the existing tech stack and its dependencies. Generative AI can help unravel this complexity by automatically analyzing legacy systems, identifying dependencies, and mapping out business processes. This comprehensive understanding reduces the risk of errors and oversights during the modernization process.
- Change Management Challenges: Resistance to change, lack of stakeholder buy-in, and cultural barriers can impede the success of modernization efforts. Generative AI can facilitate change management by providing data-driven insights, predictive analytics, and recommendations for optimizing the modernization process. By harnessing the power of AI-driven insights, organizations can navigate the complexities of change management more effectively and ensure the successful adoption of modernized systems.
Conclusion
Though a healthy skepticism is natural, acceptance of generative AI for application modernization is ascending. Businesses already use it for benefits like API discovery, consistent code generation, data handling, and more. With a plethora of benefits enabling organizations to achieve their modernization goals, generative AI is set to navigate the strategic imperative of driving sustainable growth for modern digital ecosystems.